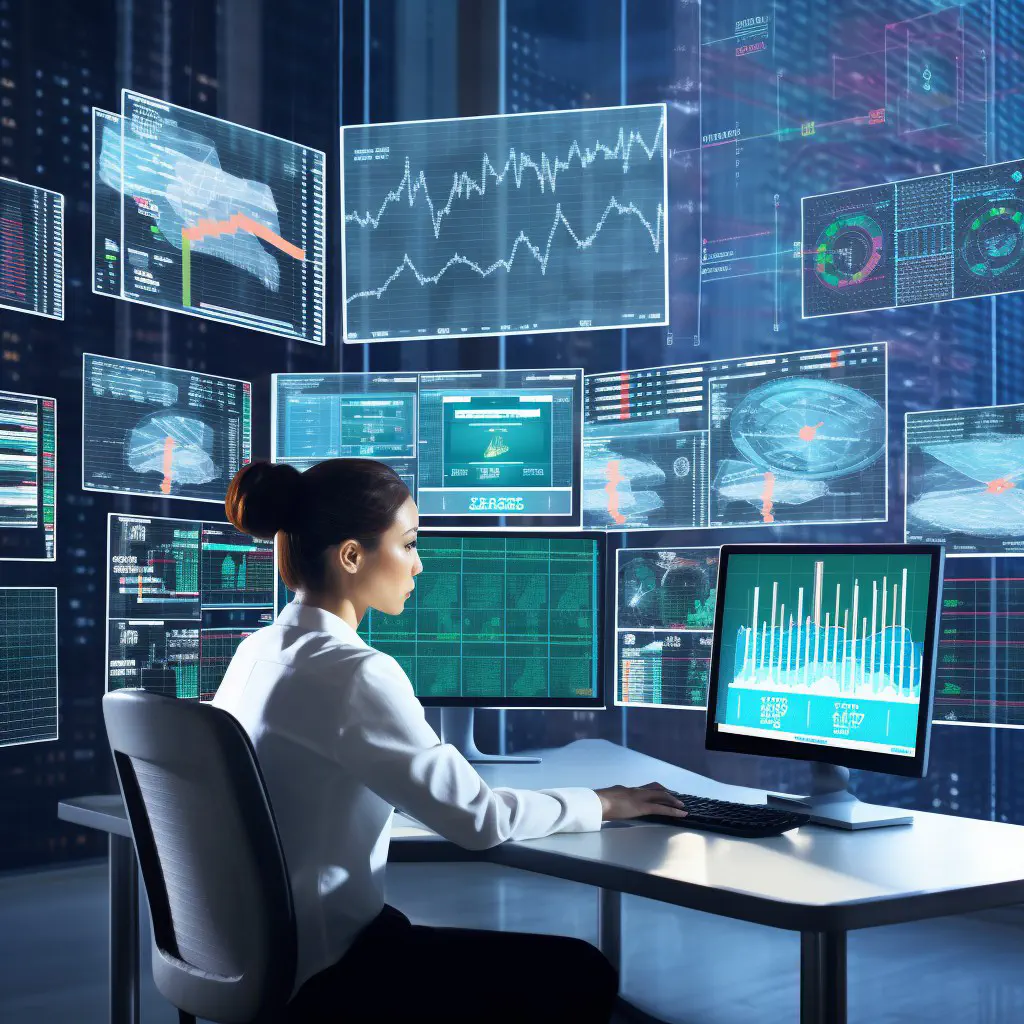
Introduction
Data analysis has become an increasingly important skill in today’s world, allowing individuals and organizations to make informed decisions using available data. With the constant flow of data coming in from various sources, it is crucial to know how to manage and analyze this data effectively. In this guide, we will cover the essential topics needed to analyze data like a pro.
Throughout the guide, we will explore the significance of collecting accurate data, the importance of cleaning data before analyzing, the benefits of data visualization, and techniques for performing effective data analysis.
By the end of this guide, you will have a better understanding of how to approach data analysis projects and create informed business decisions. Let’s get started!
Data Collection
Effective data analysis starts with collecting accurate and relevant data. Without trustworthy data, your findings will be unreliable and the insights you gain will be flawed. In this section, we will discuss the importance of data collection, methods for collecting data, and tips for ensuring data accuracy.
The Importance of Collecting Accurate Data
Collecting accurate data is essential because it forms the foundation of your analysis. Data accuracy refers to the correctness and consistency of the data. Inaccurate data can lead to flawed analysis, which can negatively impact decision-making. To ensure that your data is accurate, you need to follow a rigorous data collection process.
Methods for Collecting Data
There are various methods for collecting data, and the method you choose depends on your objectives, resources, and the type of data you need. Common methods for collecting data include:
- Surveys
- Interviews
- Focus groups
- Observation
- Secondary data sources
You need to choose the method that best suits your objectives and the type of data that you need to collect.
Tips for Ensuring Data Accuracy
To ensure data accuracy, you need to take various measures, such as:
- Defining clear and specific research objectives
- Having a well-defined sample frame
- Using reliable data collection tools and methods
- Ensuring all data is recorded correctly and completely
- Checking for outliers or errors in the data
- Performing data quality checks and cleaning
By following these steps, you can ensure that your data is accurate, reliable, and ready for analysis.
Data Cleaning
Data cleaning is an integral step in any data analysis process. It involves identifying and correcting or removing inaccuracies, inconsistencies, and errors from the collected data to ensure its quality.
Why is Data Cleaning Necessary?
Data cleaning is necessary for several reasons, including:
- Preventing errors in analysis: Analyzing dirty data can lead to incorrect conclusions and inaccurate decisions.
- Improving data quality: Dirty data can compromise the quality of data and make it unusable for analysis.
- Saving time and resources: Cleaning data upfront saves time and resources that would be spent correcting errors during analysis.
Techniques for Cleaning Data
Various techniques can be used to clean data, including:
- Removing duplicates: Duplicates can skew analysis results. They should be removed to ensure data accuracy.
- Filling in missing values: Missing values should be replaced with the mean or median of the respective column or use interpolation techniques.
- Correcting errors: Errors such as typos, incorrect formatting, and invalid values should be corrected. The use of data validation rules can help in achieving that.
- Merging datasets: When working with multiple data sources, merging datasets can help ensure consistency and accuracy.
- Normalizing data: When data is stored in different formats, normalization can help make it consistent and easier to analyze.
Importance of Data Quality for Analysis
Data quality is critical for accurate analysis. Poor data quality can lead to incorrect conclusions. A dataset with missing, inconsistent, or duplicative data could cause significant problems in the data analysis process.
Data quality assurance measures should be implemented early in the data analysis process to ensure high-quality data. Investing resources in data cleaning and quality assurance upfront saves time, minimizes errors, and leads to more accurate conclusions.
Data Visualization
Data visualization is the process of representing complex data in a visually appealing and easy-to-understand format. It plays a crucial role in data analysis, as visualizing data can help to reveal patterns, trends, and insights that may be difficult to decipher from raw data alone. Here are some reasons why you should use data visualization:
- Data exploration: Visualization can simplify the process of exploring your data, allowing you to quickly identify relationships and patterns.
- Communication: Visualizations are an effective way to communicate data insights to others, particularly those who may not have a technical background.
- Clarity: Visualizations can help to clarify complex data sets, making it easier to understand and draw conclusions from.
When it comes to creating visualizations, there are many types to choose from. Here are some of the most common:
- Bar charts and histograms: These are useful for visualizing numerical data.
- Line graphs: These are effective at displaying trends over time.
- Scatter plots: These can help to identify relationships between two variables.
- Pie charts: These are useful for showing proportions or percentages.
To create effective visualizations, here are some tips to keep in mind:
- Simplicity: Keep your visualizations simple and easy to understand.
- Accuracy: Make sure your visualizations accurately represent the data.
- Context: Provide context to your visualizations to help viewers understand what they are seeing.
- Color: Use color effectively to highlight important information.
Ultimately, the goal of data visualization is to make data accessible and understandable, so that insights can be drawn from it. By keeping these tips in mind, you can create visualizations that effectively communicate your data insights.
Data Analysis
Data analysis is the process of inspecting, cleaning, transforming, and modeling data with the aim of discovering useful information, drawing conclusions, and supporting decision-making. There are different types of data analysis, including descriptive analysis, diagnostic analysis, predictive analysis, and prescriptive analysis.
Descriptive Analysis
Descriptive analysis involves summarizing and describing the main features of a dataset. It involves exploring patterns, frequencies, and trends within the data. Descriptive analysis is often used to gain an initial understanding of the data and to identify any potential outliers or anomalies.
Diagnostic Analysis
Diagnostic analysis involves investigating the underlying causes of a problem or issue. It is used to determine what factors or variables may be contributing to a specific outcome. For example, diagnostic analysis can help identify the root cause of a decrease in sales or an increase in customer complaints.
Predictive Analysis
Predictive analysis involves using statistical models and machine learning algorithms to make predictions or forecasts based on historical data. This type of analysis is often used to identify potential future trends or outcomes. It can help organizations make decisions based on the likelihood of a specific outcome.
Prescriptive Analysis
Prescriptive analysis involves recommending actions or decisions based on the results of data analysis. It is used to determine the best course of action to take in a given situation. Prescriptive analysis often involves identifying multiple potential outcomes and selecting the one that is most beneficial.
Choosing the right type of data analysis for your data depends on the specific problem or issue you are trying to address. It also requires a good understanding of the available data, the underlying business context, and the tools and methods available.
Techniques for Performing Analysis
There are many techniques that can be used to perform data analysis. These include statistical analysis, data mining, machine learning, and text analytics. Statistical analysis involves using mathematical techniques to identify patterns and trends in data. Data mining involves using algorithms to discover patterns and relationships in large datasets. Machine learning involves building models and algorithms that can learn from data and make predictions. Text analytics involves analyzing text data to identify patterns, sentiments, and themes.
When performing data analysis, it is important to use the right techniques for the specific type of data and problem being addressed. It is also important to ensure data quality and accuracy, as well as to document all steps of the analysis process for transparency and reproducibility.
In conclusion, mastering data analysis requires a combination of technical skills, domain knowledge, and critical thinking. By following the right processes and using the right tools and techniques, anyone can become a data analysis pro.
Conclusion
After going through this comprehensive guide, you are now equipped with the knowledge to analyze data like a pro. Let’s recap the key takeaways from each section.
In the Introduction, we explained the importance of data analysis and gave a brief overview of the topics covered in the guide.
The Data Collection section emphasized the need for accurate data collection and discussed methods for achieving this. We also provided tips on how to ensure data accuracy.
For Data Cleaning, we explained the importance of cleaning data and provided techniques for cleaning it. We highlighted the importance of data quality for analysis.
In Data Visualization, we discussed the reasons for using data visualization and provided information on common types of visualizations. We also gave tips on how to create effective visualizations.
The Data Analysis section explained the different types of data analysis and provided techniques for performing analysis. We also emphasized the importance of choosing the right analysis for your data.
In conclusion, mastering the art of data analysis is essential for any professional with the responsibility of interpreting large amounts of data. Use the techniques provided in this guide to deliver data-driven insights. Remember that collecting accurate data, cleaning it properly, visualizing it, and analyzing it using the right techniques are the keys to becoming an expert data analyst.