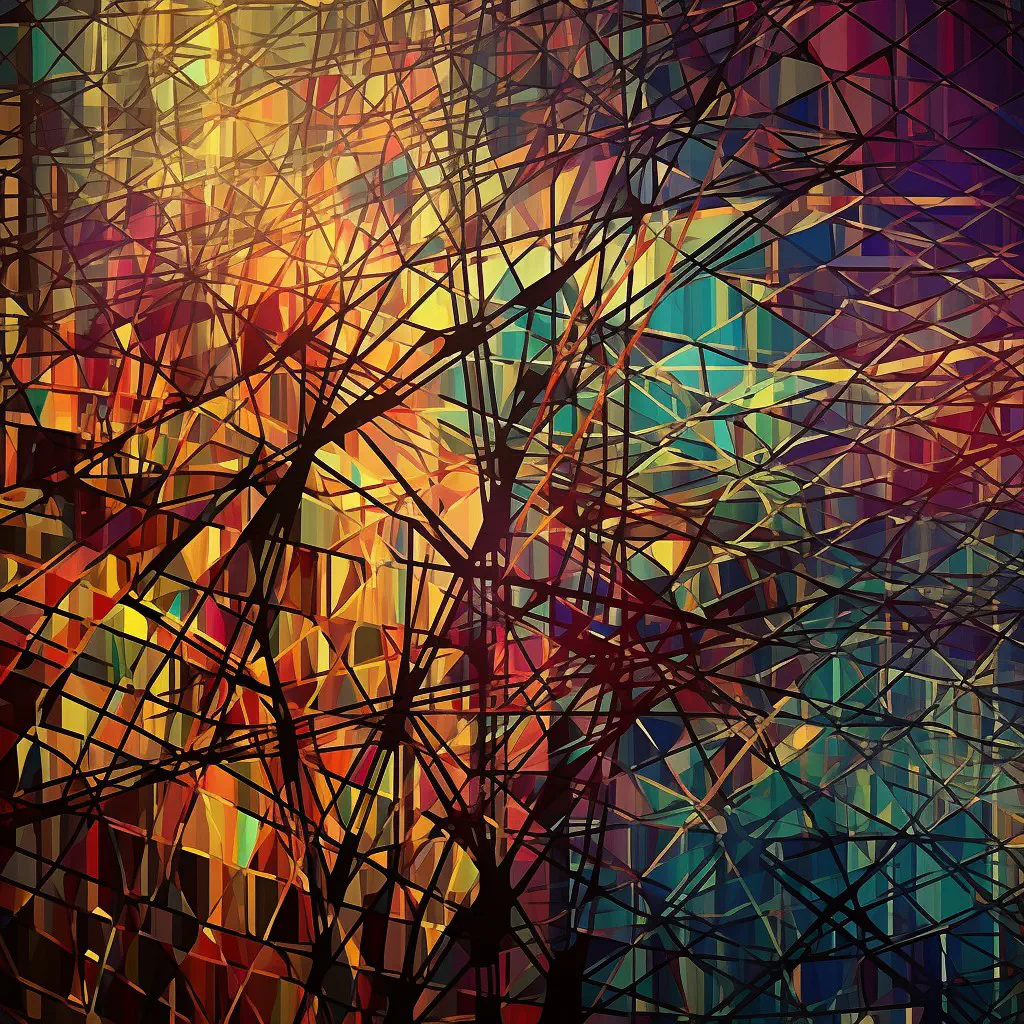
Introduction
Pattern recognition is a crucial aspect of artificial intelligence (AI) development that enables machines to identify and interpret patterns in data. This ability plays a significant role in advancing problem-solving and decision-making capabilities of AI systems, leading to groundbreaking applications in various fields.
“Pattern recognition empowers machines to make sense of complex data by identifying recurring patterns and extracting meaningful insights.”
By analyzing data patterns, machines can detect similarities, understand relationships, and predict future outcomes. This capability has revolutionized areas such as image recognition, speech recognition, and natural language processing, opening up new possibilities for innovation and transforming industries.
In order to recognize patterns, machines are trained using large datasets that contain examples of the patterns they are supposed to identify. Through a process called machine learning, AI models are developed and trained to learn from these examples, allowing them to generalize their understanding and recognize patterns in new and unseen data.
Pattern recognition is an integral part of AI advancement and has witnessed significant progress over the years, resulting in remarkable breakthroughs and milestones. Let’s dive deeper into the concept of pattern recognition and explore its applications and implications in the world of AI.
Understanding Pattern Recognition
Pattern recognition is a fundamental concept in the field of artificial intelligence (AI) that enables machines to learn and identify patterns in data. It is the process by which systems recognize regularities or similarities in information and make accurate predictions or classifications based on those patterns.
Application in Various Fields
Pattern recognition finds applications in various fields, revolutionizing industries and improving our daily lives. Some notable examples include:
-
Image Recognition: Pattern recognition has greatly advanced image processing and computer vision. AI systems can accurately identify objects, faces, and other features in images, enabling applications like facial recognition, object detection, and autonomous vehicles.
-
Speech Recognition: Pattern recognition algorithms have enabled significant advancements in speech recognition technology. AI systems can now accurately transcribe audio data, convert speech to text, and recognize voice commands, leading to applications like virtual assistants, transcription services, and automated voice response systems.
-
Natural Language Processing: Pattern recognition plays a pivotal role in natural language processing (NLP). AI models can understand and analyze human language patterns, enabling tasks such as sentiment analysis, language translation, chatbots, and text summarization.
Training Machines to Recognize Patterns
Machines learn to recognize patterns through a process known as machine learning. The training process involves providing the AI system with a large dataset containing examples of the patterns to be recognized. The system then analyzes and extracts features from the data, identifying relationships between inputs and outputs. Through iterative learning and optimization algorithms, the machine adjusts its internal parameters to improve its pattern recognition accuracy.
There are various approaches to pattern recognition in machine learning, such as supervised learning, unsupervised learning, and reinforcement learning. Each approach has its own advantages and is suited for different problem domains.
Supervised learning involves training the machine using labeled examples, where the desired output is provided for each input. Unsupervised learning, on the other hand, involves discovering patterns in data without any explicit guidance. Reinforcement learning focuses on training agents to interact with an environment and learn from the consequences of their actions, incorporating patterns in decision-making processes.
Pattern recognition algorithms used in machine learning include neural networks, support vector machines, decision trees, and hidden Markov models, among others. These algorithms enable machines to detect and generalize patterns from complex datasets, making them valuable tools for AI development.
In conclusion, understanding pattern recognition is crucial for grasping the capabilities of AI systems. It is the underlying mechanism that allows machines to recognize patterns, leading to significant advancements in image recognition, speech recognition, and natural language processing. Through machine learning algorithms, machines can continually improve their pattern recognition abilities, enabling them to make accurate predictions and classifications.
Advancements in Pattern Recognition
Historical Development of Pattern Recognition in AI
The field of Pattern Recognition has witnessed significant advancements over the years, playing a crucial role in the growth of Artificial Intelligence. From its inception to the modern era, various breakthroughs and milestones have propelled Pattern Recognition forward.
Early Beginnings
Pattern Recognition in AI can be traced back to the 1950s when researchers started exploring ways to develop machines capable of understanding and identifying patterns. The initial focus was primarily on simple patterns, such as recognizing handwritten characters or basic shapes.
Machine Learning and Pattern Recognition
One of the key advancements in Pattern Recognition is the integration of Machine Learning techniques. In the 1980s and 1990s, researchers began employing algorithms that allowed machines to learn from data and improve pattern recognition capabilities. This shift enabled machines to handle more complex patterns and achieve higher accuracy.
Deep Learning and Neural Networks
The advent of Deep Learning revolutionized Pattern Recognition in AI. Deep Learning algorithms, particularly neural networks, became pivotal in unlocking new levels of pattern identification. Neural networks, through multiple layers of interconnected nodes, can autonomously learn to recognize patterns by extracting key features from large datasets. This breakthrough has led to remarkable progress in image recognition, natural language processing, and speech recognition.
Examples of Real-World Applications
Image Recognition
Pattern Recognition has been extensively utilized in image recognition applications. Deep Learning-enabled algorithms can now accurately identify objects, faces, and even specific details within images. This has paved the way for various applications, such as self-driving cars, surveillance systems, and medical imaging diagnostics.
Speech Recognition
Speech recognition technology heavily relies on Pattern Recognition to convert spoken language into written text. Advanced algorithms analyze speech patterns and identify phonemes, words, and sentences, enabling accurate transcription and voice control systems like virtual assistants.
Natural Language Processing
Pattern Recognition methods are fundamental in Natural Language Processing (NLP) tasks. Through techniques like sentiment analysis and named entity recognition, machines can understand and analyze textual data, leading to applications such as automated chatbots, language translation, and information retrieval systems.
Realizing the Potential
Pattern Recognition has brought about tremendous benefits to AI systems, enhancing their problem-solving and decision-making capabilities.
Efficient Data Analysis
By recognizing patterns, AI systems can quickly analyze vast amounts of data and extract valuable insights. This leads to more efficient decision-making and identification of trends that might otherwise remain hidden.
Automation and Personalization
Pattern Recognition-driven AI systems enable automation across various fields. By recognizing patterns in tasks or behaviors, machines can automate repetitive processes and personalize user experiences. For example, e-commerce platforms leverage Pattern Recognition to offer personalized product recommendations based on users’ preferences and purchase history.
Fraud Detection and Security
Pattern Recognition plays a crucial role in fraud detection and security systems. By identifying unusual patterns or anomalies within data, AI algorithms can identify potential threats, such as fraudulent transactions or security breaches.
Limitations and Challenges
While there have been substantial advancements, Pattern Recognition also faces certain challenges and limitations in AI.
Data Dependency
Pattern Recognition algorithms heavily rely on high-quality training data. Insufficient or biased datasets can lead to inaccurate pattern identification or biased decision-making. Acquiring and labeling large-scale datasets for training remains a challenge, particularly in niche domains.
Computational Requirements
The computational resources required for training and deploying Pattern Recognition models can be extensive. Deep Learning models, for instance, often demand significant computing power and memory. Meeting these requirements can be a hindrance, especially for resource-constrained applications or devices.
Ethical Considerations
Pattern Recognition technologies raise ethical concerns, especially in areas like facial recognition and privacy. The potential misuse of pattern recognition systems, such as surveillance technology, needs careful oversight and regulation to ensure they respect individuals’ privacy and human rights.
The Future of Pattern Recognition
The future of Pattern Recognition in AI holds immense possibilities for further advancements and improvements.
Explainable AI
Efforts are being made to develop more explainable AI systems that can provide insights into the underlying patterns recognized. Understanding the decision-making process of AI models can facilitate trust and transparency, paving the way for their wider adoption.
Cross-Domain Applications
Pattern Recognition is now being explored in the context of cross-domain applications. This involves transferring knowledge and patterns recognized in one domain to solve problems in entirely different domains. Such developments could lead to breakthroughs in multidisciplinary fields and enable rapid problem-solving across industries.
Integration with Edge Computing
Advancements in hardware and edge computing are enabling Pattern Recognition models to be deployed directly onto devices. This decentralization can enhance real-time pattern recognition capabilities and reduce the dependency on cloud-based processing, ultimately enabling applications such as real-time object identification on IoT devices.
In conclusion, Pattern Recognition is a critical component of AI development, enabling machines to identify and interpret complex patterns from various data sources. The continuous advancements in Pattern Recognition techniques hold great promise for the future of AI, making it an exciting and transformative field of study. As researchers and practitioners explore new frontiers, it is crucial to address the challenges and ethical considerations associated with Pattern Recognition technologies to harness their potential for the benefit of society.
Benefits of Pattern Recognition in AI
Pattern recognition plays a crucial role in the development of artificial intelligence (AI) systems, offering a wide range of benefits. Let’s explore some of the key advantages of pattern recognition-driven AI:
Enhanced Problem-Solving Abilities
By leveraging pattern recognition, AI systems can efficiently analyze large datasets and identify recurring patterns or trends. This capability enables them to solve complex problems quickly and accurately. For example, in finance, AI algorithms can recognize patterns in stock market data to identify profitable investment opportunities. Similarly, in healthcare, AI-powered pattern recognition algorithms can detect patterns in patient symptoms and medical records to assist in diagnosis and treatment plans.
“Pattern recognition-powered AI systems excel in identifying complex patterns, allowing for enhanced problem-solving abilities across industries.”
Improved Decision-Making Capabilities
Pattern recognition in AI also contributes to improved decision-making. AI algorithms can analyze patterns in data from various sources and provide valuable insights to support decision-making processes. For instance, in business, AI-powered systems can recognize patterns in customer behavior and preferences, helping companies make informed marketing and product development decisions. In autonomous vehicles, pattern recognition algorithms enable the vehicle to interpret and respond to traffic patterns, enhancing safety and efficiency.
Automation and Efficiency
Pattern recognition allows AI systems to automate complex tasks and processes, leading to increased efficiency and productivity. By recognizing patterns in repetitive tasks, AI algorithms can streamline workflows, reduce the need for human intervention, and save time and resources. For instance, in manufacturing, pattern recognition algorithms can detect defects or anomalies in products at high speeds, helping ensure quality control. In customer service, AI-powered chatbots equipped with pattern recognition abilities can provide personalized responses and support to users, improving overall customer experience.
Real-Life Examples
The benefits of pattern recognition-driven AI are witnessed in numerous real-life applications. One such example is image recognition technology used in security systems. AI algorithms can identify patterns in images or videos to detect suspicious activities, making it easier to prevent crimes or respond promptly. Another example is speech recognition technology, employed in voice assistants like Siri or Google Assistant. Through pattern recognition, these AI systems can accurately understand and interpret spoken language, enabling users to interact with devices using voice commands.
In the field of natural language processing (NLP), pattern recognition enables AI systems to comprehend and generate human-like language. This has led to advancements in machine translation, sentiment analysis, and chatbot interactions, further improving communication between humans and machines.
“Pattern recognition-driven AI systems have a profound impact on various industries, enhancing problem-solving, decision-making, and automating tasks for increased efficiency.”
In conclusion, pattern recognition is a fundamental component of AI, offering significant benefits across different domains. From problem-solving and decision-making to automation and efficiency, AI systems empowered by pattern recognition play a vital role in transforming industries and enhancing overall user experiences. As technology advances, it is crucial to further explore and embrace the capabilities of pattern recognition in AI, shaping a future where machines can intuitively understand and respond to complex patterns and behaviors.
Challenges and Limitations
Challenges faced in implementing Pattern Recognition
Implementing Pattern Recognition in AI systems comes with its own set of challenges. Some of the key challenges include:
-
Data availability and quality: Pattern Recognition algorithms heavily rely on large amounts of high-quality data for training. However, obtaining such data can be challenging in certain domains, especially in fields where labeled data is scarce or expensive to collect.
-
Algorithm complexity: Pattern Recognition algorithms can be complex and require significant computational resources. Implementing and optimizing these algorithms for real-time applications can be a challenging task, particularly for resource-constrained systems.
-
Feature selection and representation: Choosing the right set of features and representing them appropriately is crucial for effective pattern recognition. However, selecting meaningful features that capture the essential characteristics of the data can be a challenging task, especially in complex and high-dimensional datasets.
-
Overfitting and generalization: Overfitting occurs when a pattern recognition model memorizes the training data too well and fails to generalize to unseen data. Balancing the model’s capability to capture complex patterns while avoiding overfitting is a challenging task that requires careful consideration.
Limitations and drawbacks of Pattern Recognition in AI
While Pattern Recognition is a powerful tool in AI development, it does have certain limitations and potential drawbacks:
-
Limited interpretability: Deep learning approaches, which are often used for pattern recognition, are notorious for their lack of interpretability. Understanding why a model made a certain decision or recognizing the exact features it used to identify a pattern can be difficult, limiting our ability to trust and explain AI systems.
-
Domain-specific constraints: Pattern Recognition systems may struggle to handle domains where patterns are not well-defined or where the input data is noisy or ambiguous. These challenges are particularly pronounced in tasks such as anomaly detection, where deviations from expected patterns may signify important events.
-
Vulnerability to adversarial attacks: Pattern Recognition models can be vulnerable to adversarial attacks, where malicious actors deliberately manipulate the input data to mislead the model’s predictions. These attacks can have severe consequences in fields such as computer vision and natural language processing, highlighting the need for robust defenses.
Potential risks and ethical concerns pertaining to Pattern Recognition technologies
As Pattern Recognition technologies continue to advance, it is important to address the potential risks and ethical concerns associated with their usage:
-
Bias and fairness: Pattern Recognition algorithms can inadvertently perpetuate existing biases present in the training data. If the training data is biased or not representative of the real-world diversity, the resulting AI systems can exhibit discriminatory behavior, potentially amplifying biases and causing unfair decision-making.
-
Privacy and security: Pattern Recognition systems often require access to sensitive data, such as personal images, voice recordings, or text messages. Ensuring the privacy and security of this data is of utmost importance to prevent unauthorized access or misuse.
-
Automation and job displacement: The increasing adoption of Pattern Recognition-driven AI systems has the potential to automate certain tasks, leading to job displacement in some industries. It is crucial to consider the socio-economic impacts of such automation and develop strategies to mitigate any adverse consequences.
-
Legal and regulatory challenges: The use of Pattern Recognition technologies raises legal and regulatory challenges. Ensuring compliance with existing laws, such as data protection and privacy regulations, and developing robust ethical frameworks to govern their use is essential to prevent misuse and protect the rights of individuals.
In conclusion, while Pattern Recognition holds great potential in AI development, it is essential to address the challenges, limitations, and ethical concerns associated with its implementation. By addressing these issues, we can harness the power of Pattern Recognition technologies to create AI systems that are reliable, interpretable, and fair, ensuring their positive impact on various domains and society as a whole.
Future Prospects
Advancements in Pattern Recognition Algorithms
With the rapid development of AI technologies, we can expect significant advancements in pattern recognition algorithms. Researchers are continuously finding new ways to improve the efficiency and accuracy of pattern recognition models. One area of focus is the development of deep learning algorithms that can automatically learn and extract complex patterns from large datasets. These advancements will enable AI systems to recognize patterns with higher precision and make more informed decisions.
Integration of Pattern Recognition with Other AI Technologies
In the future, we can anticipate the integration of pattern recognition with other AI technologies, such as natural language processing, machine vision, and robotics. By combining these capabilities, AI systems will be able to understand and interpret patterns in different modalities, leading to more comprehensive analysis and decision-making. For example, a robot equipped with pattern recognition and computer vision can identify objects in its surroundings and recognize patterns associated with human actions, enabling it to interact intelligently with humans.
Pattern Recognition in Healthcare and Medicine
Pattern recognition has promising prospects in healthcare and medicine. By analyzing large amounts of medical data, AI systems can identify subtle patterns and correlations that might go unnoticed by human physicians. This could lead to earlier and more accurate diagnoses, personalized treatment plans, and improved patient outcomes. For instance, AI algorithms trained on medical images can detect patterns indicative of diseases, such as cancers or abnormalities, assisting radiologists in their diagnosis.
Pattern Recognition in Cybersecurity
As cyber threats become more sophisticated, there is a growing need for advanced pattern recognition techniques in cybersecurity. Machine learning algorithms can analyze network traffic patterns and detect anomalies that may indicate a potential security breach or malicious activity. By continuously learning from evolving patterns, AI systems can adapt and strengthen their defenses against emerging cybersecurity threats. This proactive approach will help in identifying and mitigating security risks before they cause significant damage.
Ethical Considerations and Regulation
As pattern recognition technologies become more pervasive, there will be a need for careful ethical considerations and regulation to address concerns related to privacy, bias, and fairness. AI systems built on pattern recognition algorithms may unintentionally learn biases from the training data, leading to discriminatory decisions or perpetuating existing social inequalities. It will be essential to develop frameworks to ensure transparency, accountability, and fairness in the deployment of pattern recognition technologies.
Collaboration between Human and AI
The future of pattern recognition in AI lies in a collaborative approach between humans and machines. While machines excel at recognizing and analyzing patterns, human expertise and contextual understanding are invaluable in interpreting and making sense of the patterns identified. By harnessing the strengths of both humans and AI, we can achieve better problem-solving, decision-making, and innovation. This collaborative partnership will facilitate the development of AI systems that augment human capabilities and empower individuals across various domains.
In conclusion, the future prospects of pattern recognition in AI are promising. Advancements in algorithms, integration with other AI technologies, and its application in healthcare, cybersecurity, and various other fields hold immense potential. However, it is crucial to address ethical concerns and ensure responsible development and deployment of pattern recognition technologies. By embracing pattern recognition and its future advancements, we can unlock new possibilities and shape a more intelligent and efficient future powered by AI.
Conclusion
Throughout this blog, we have explored the significance of pattern recognition in AI development. We have seen how machines can identify patterns and how this capability enhances their problem-solving and decision-making abilities.
Pattern recognition plays a crucial role in various fields such as image recognition, speech recognition, and natural language processing. These applications have revolutionized industries such as healthcare, finance, and manufacturing, with their ability to analyze vast amounts of data and provide valuable insights.
The historical development of pattern recognition in AI has witnessed significant breakthroughs and key milestones. From early research in the field to the development of complex algorithms and deep learning techniques, pattern recognition has advanced tremendously. Real-world applications like facial recognition, voice assistants, and autonomous vehicles serve as testament to the effectiveness of pattern recognition-driven AI systems.
The benefits of pattern recognition in AI are manifold. By recognizing patterns, AI systems can quickly identify anomalies, detect fraud, predict future outcomes, and make informed decisions. This ability to process and analyze patterns enables AI to uncover hidden insights and provide valuable recommendations, driving efficiency and innovation across industries.
However, implementing pattern recognition also comes with its share of challenges and limitations. The complexity of developing accurate and robust pattern recognition algorithms requires substantial computational power and extensive training data. Additionally, ethical concerns, such as privacy infringement and biased decision-making, need to be addressed to ensure responsible usage of pattern recognition technologies.
Looking ahead, the future of pattern recognition in AI development is promising. As computing power continues to grow and more data becomes available, AI systems will become increasingly adept at recognizing patterns and making sense of complex information. Enhancements such as explainable AI, which provides transparency in decision-making processes, will further improve the adoption and trust in pattern recognition technologies.
In conclusion, pattern recognition is a fundamental aspect of AI development, enabling machines to understand, analyze, and interpret the world around us. Its impact on problem-solving and decision-making capabilities is profound, revolutionizing industries and paving the way for a future where AI-driven systems become an integral part of our daily lives. As pattern recognition technologies continue to advance, we encourage readers to explore and embrace their potential, as they hold the key to unlocking transformative possibilities in AI.