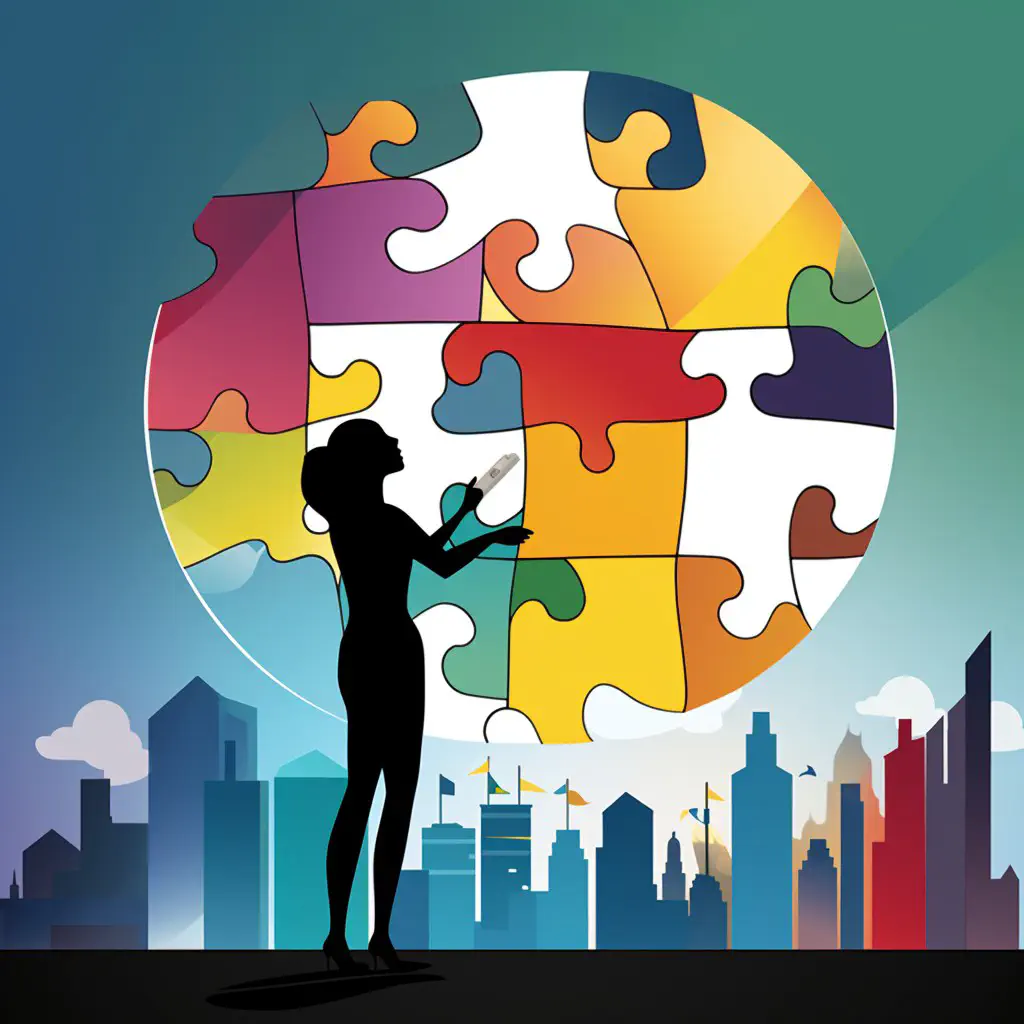
Introduction
Contextual analysis is a powerful tool for gaining insight into various phenomena. Whether it’s understanding the political climate or discovering consumer behavior patterns, contextual analysis can provide valuable information. However, as with any analytical process, there are challenges that need to be overcome. In this article, we will explore these challenges and the best practices for overcoming them.
Data is the foundation of contextual analysis, and data quality is paramount to ensuring reliable and accurate results. Poor data quality can lead to misleading conclusions, which can be detrimental to decision-making. We will discuss common issues with data quality and how to ensure the data used is of the highest quality.
In addition to data quality, data diversity is another important consideration in contextual analysis. Incorporating diverse data sources can lead to more comprehensive and nuanced insights. We will dive into the challenges of data diversity and best practices for incorporating various data sources.
Lastly, data relevance is key to the success of contextual analysis. With so much data available, it can be challenging to determine which data is relevant and which is not. We will examine common issues surrounding data relevance and the best approaches for filtering data to ensure the most critical intel is being utilized.
Overall, this article aims to highlight the importance of overcoming challenges in contextual analysis and provide practical solutions for doing so. By improving the data quality, data diversity, and data relevance in contextual analysis, we can generate more insightful results and make better-informed decisions.
Data Quality
Poor quality data can lead to inaccurate and unreliable contextual analysis. Therefore, it is crucial to ensure the quality of data used in contextual analysis. Some common issues with data quality include inaccurate or incomplete data, data duplication, and data inconsistency. To overcome these challenges, some solutions include:
- Conducting data audits: Regularly audit the data used in contextual analysis to identify any inaccuracies, incompleteness, or inconsistencies.
- Ensuring data accuracy: Use proper data validation techniques to ensure the accuracy of the data used in contextual analysis.
- Managing data duplication: Ensure that data duplication is effectively managed to ensure the accuracy and consistency of the data.
- Data cleansing: Engage in data cleansing to remove any outdated, inconsistent, or irrelevant data from the dataset.
To ensure data quality in contextual analysis, some best practices include:
- Developing data quality standards: Establish clear data quality standards that identify what constitutes high-quality data.
- Regular data backups: Regularly back up data to ensure the availability of data for further analysis.
- Proper data governance practices: Establish proper data governance practices to ensure the high quality and accuracy of data used in contextual analysis.
- Providing Data Quality Training: Providing training to ensure that all members of the team are aware of the significance of data quality and compliance with data quality standards.
With these best practices in place, it will be easier to overcome common challenges relating to data quality in contextual analysis and produce reliable results.
Data Diversity
In contextual analysis, it is important to consider the diversity of data sources to ensure a comprehensive and accurate understanding of the context. Without diverse data, analysts risk missing important perspectives and biases in their analysis.
Common issues with data diversity and their solutions
Some common issues with data diversity include:
- Limited access to diverse data sources
- Difficulty in integrating and analyzing diverse data sources
- Limited understanding of the cultural and linguistic nuances of diverse data sources
To address these issues, it is important to:
- Identify and source diverse data sets from a variety of domains and industries
- Use tools and technologies to harmonize, integrate and analyze diverse data sources
- Work with experts in the field to understand the cultural and linguistic nuances of diverse data sources
Best practices for incorporating diverse data sources in contextual analysis
To incorporate diverse data sources in contextual analysis, analysts should follow these best practices:
- Define and prioritize the types of data that will be used in the analysis
- Source data from different domains, industries, and cultures
- Evaluate the quality and reliability of the data before including it in the analysis
- Use tools and technologies to integrate data from different sources
- Work with experts in the field to analyze and interpret data from different sources
Incorporating diverse data sources into contextual analysis not only leads to a more accurate understanding of the context but also helps to identify biases and assumptions that may have gone unnoticed otherwise.
Data Relevance
In contextual analysis, the relevance of data is crucial in ensuring accurate and meaningful results. Relevant data provides insight into the specific contexts being studied and allows for better analysis. However, it is common for irrelevant data to be included in analysis, and this can negatively impact the accuracy of results.
Common issues with data relevance and their solutions
One common issue with data relevance is the inclusion of data that is not specific to the context being studied. For example, in an analysis of customer behavior for a specific product, irrelevant data about unrelated products may be included. To solve this, it is important to clearly define the context of the analysis and filter out any irrelevant data. This can be achieved by using specific keywords and categories to identify relevant data sources.
Another issue is over-reliance on a single data source that may not accurately represent the context being studied. To overcome this, it is important to incorporate multiple data sources that provide different perspectives. This can include qualitative data, such as surveys or interviews, which can provide insight into the thoughts and opinions of individuals.
Best practices for selecting and filtering relevant data for contextual analysis
To ensure relevance in contextual analysis, it is important to follow certain best practices, including:
- Clearly defining the context and scope of the analysis
- Identifying and using specific keywords and categories to filter out irrelevant data
- Incorporating multiple data sources from different perspectives
- Regularly reviewing and updating data sources to ensure their continued relevance
- Using data visualization tools to identify patterns and trends in the data
By implementing these best practices, contextual analysis can provide meaningful and accurate insights into the specific contexts being studied.
Conclusion
In conclusion, contextual analysis is a critical aspect of research and analysis in various fields. However, as we have explored, it comes with its fair share of challenges that can limit its effectiveness. We have looked at the importance of data quality, diversity, and relevance in contextual analysis, and the common issues associated with each.
To improve contextual analysis processes, it is essential to implement best practices for ensuring data quality, incorporating diverse data sources, and selecting and filtering relevant data. Teams should also invest in tools and technologies that can help reduce the impact of these challenges.
Ultimately, continued efforts to address these challenges and improve contextual analysis processes will help us gain better insights, make informed decisions, and achieve more accurate results. It is up to all of us to take action and ensure that we are leveraging the full potential of contextual analysis to drive success in our respective fields.